5 Real World Examples of Data Science Roles
1. Retail
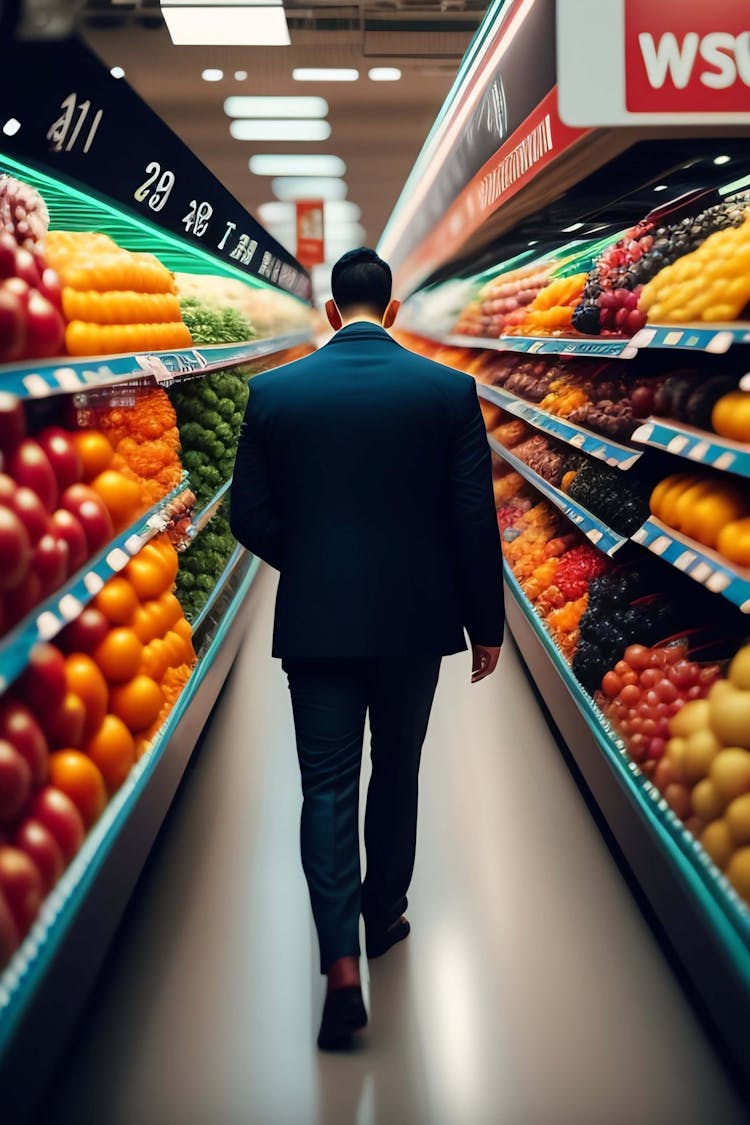
One example of a case study in data science is the analysis of customer data by a retail company. The company collects data on customer purchases, demographics, and behavior, and uses data science techniques to understand and analyze this data.
One of the goals of the analysis is to identify patterns and trends in the data that can help the company better understand its customers and improve its business operations. For example, the analysis might reveal that a certain group of customers is more likely to make large purchases, or that a particular type of product is more popular among certain age groups.
Based on these insights, the company can take action to improve its marketing and sales efforts, such as targeting specific customer segments with personalized promotions or developing new products that are likely to be popular with its customers.
The data science analysis can also help the company identify potential issues or risks, such as trends in customer behavior that may indicate a decline in customer satisfaction. By addressing these issues, the company can improve its customer retention and loyalty, and ultimately grow its business.
Overall, this case study shows how data science can be used to analyze and interpret large amounts of data and to extract valuable insights that can help businesses improve their operations and grow.
2. Agriculture
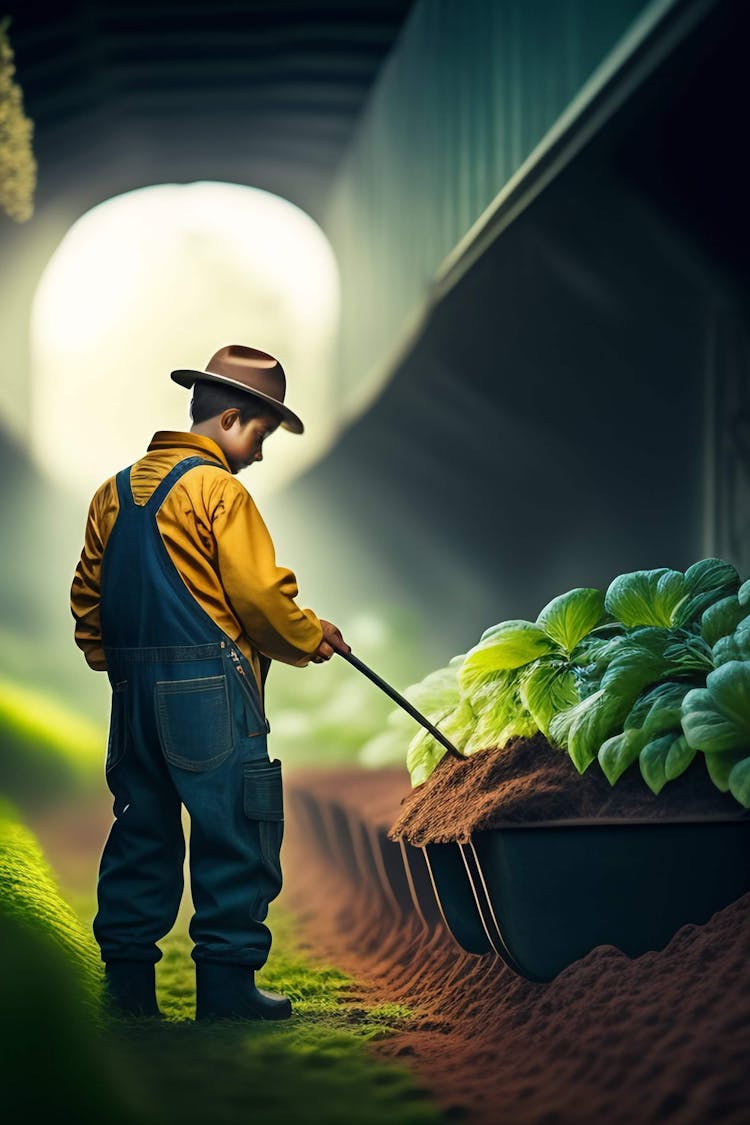
One example of a case study in data science in the agriculture field is the use of machine learning algorithms to predict crop yields. In this scenario, a farmer collects data on a variety of factors that can impact crop yields, such as weather conditions, soil type, and the type of seed used.
The farmer then uses machine learning algorithms to analyze this data and develop a model that can predict the expected yield of a particular crop, based on the available data. The model is trained on a large dataset of historical crop yield data, and is tested and refined to ensure that it is accurate and reliable.
Once the model is finalized, the farmer can use it to make more informed decisions about planting and harvesting, such as deciding which crops to plant and when to harvest them. This can help the farmer optimize the use of resources, such as land and water, and maximize the overall yield of their crops.
In addition, the farmer can also use the model to identify potential issues or risks, such as the likelihood of a crop failure due to adverse weather conditions. By addressing these issues, the farmer can reduce their exposure to risk and improve the overall success of their farming operations.
Overall, this case study shows how data science and machine learning can be used in the agriculture field to help farmers make more informed and efficient decisions, and to improve the success of their operations.
3. Healthcare
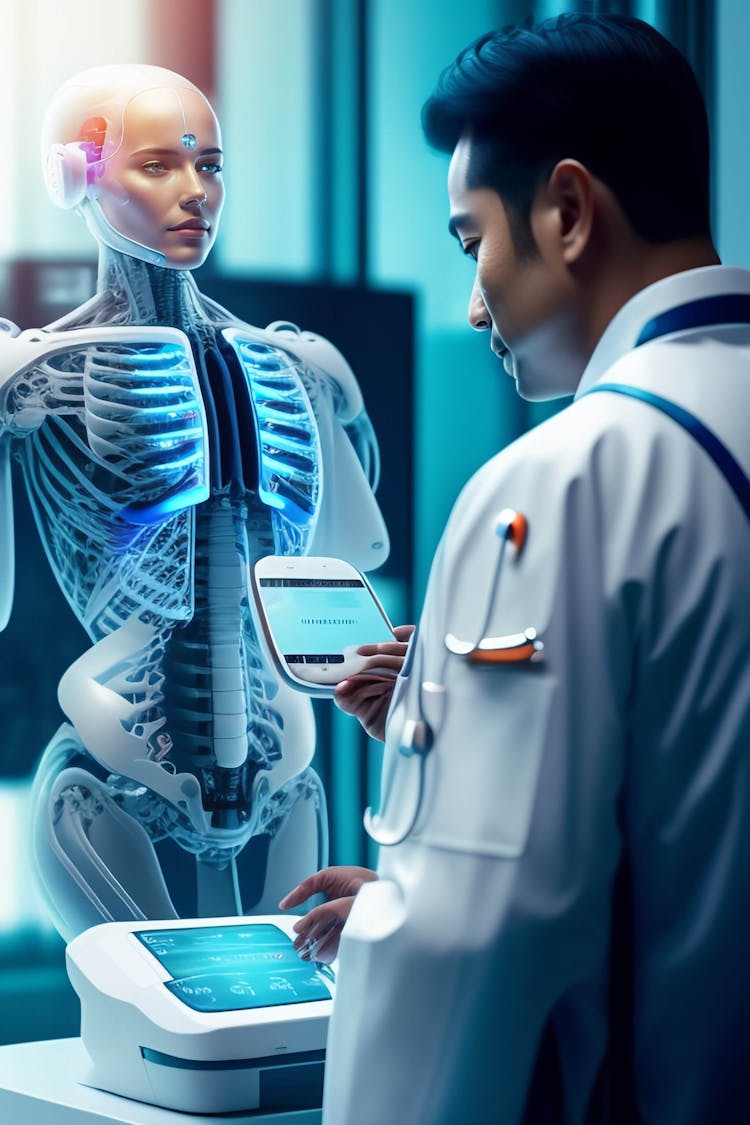
One example of a case study in data science in the healthcare field is the use of machine learning algorithms to diagnose medical conditions. In this scenario, a healthcare provider collects data on patients, including their medical history, symptoms, and test results.
The provider then uses machine learning algorithms to analyze this data and develop a model that can predict the likelihood of a particular medical condition, based on the available data. The model is trained on a large dataset of historical patient data and is tested and refined to ensure that it is accurate and reliable.
Once the model is finalized, the provider can use it to assist with the diagnosis of patients. For example, the model can help to identify potential medical conditions that may not be immediately apparent based on a patient's symptoms alone. This can help the provider to make more accurate and timely diagnoses, and to provide more effective treatment for their patients.
In addition, the provider can also use the model to identify potential risks or issues, such as the likelihood of a patient developing a particular medical condition. By addressing these issues, the provider can help to prevent or reduce the occurrence of certain medical conditions, and improve the overall health and well-being of their patients.
Overall, this case study shows how data science and machine learning can be used in the healthcare field to improve the accuracy and effectiveness of medical diagnoses and to help providers provide better care for their patients.
4. Transport
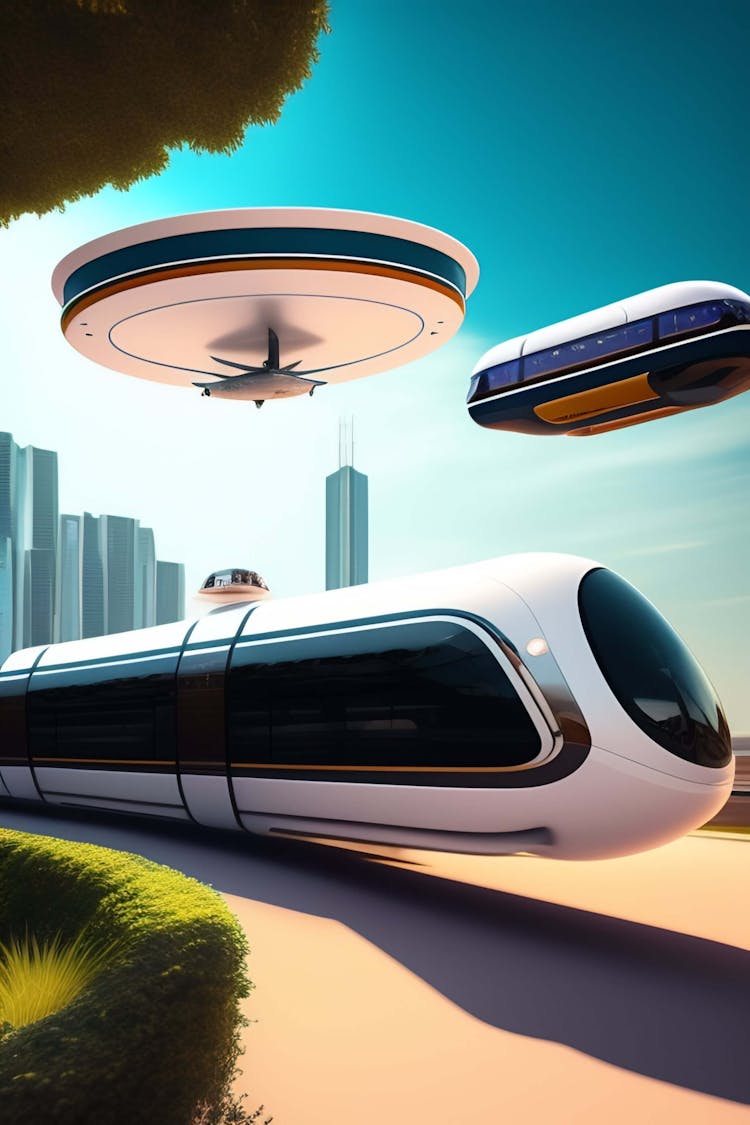
One example of a case study in data science in the transport field is the use of predictive analytics to improve the efficiency of public transportation. In this scenario, a public transportation agency collects data on the routes, schedules, and ridership of its buses and trains.
The agency then uses predictive analytics algorithms to analyze this data and develop a model that can predict the demand for public transportation at different times and locations. The model is trained on a large dataset of historical ridership data, and is tested and refined to ensure that it is accurate and reliable.
Once the model is finalized, the agency can use it to make more informed decisions about the deployment of its buses and trains. For example, the model can help the agency to identify areas where there is a high demand for public transportation, and to adjust the routes and schedules of its buses and trains accordingly. This can help the agency to improve the efficiency and reliability of its services, and to provide better service to its riders.
In addition, the agency can also use the model to identify potential issues or risks, such as potential delays or disruptions to its services. By addressing these issues, the agency can reduce the likelihood of such events occurring, and improve the overall performance of its public transportation system.
Overall, this case study shows how data science and predictive analytics can be used in the transport field to improve the efficiency and reliability of public transportation services, and to provide better service to riders.
5. e-Commerce
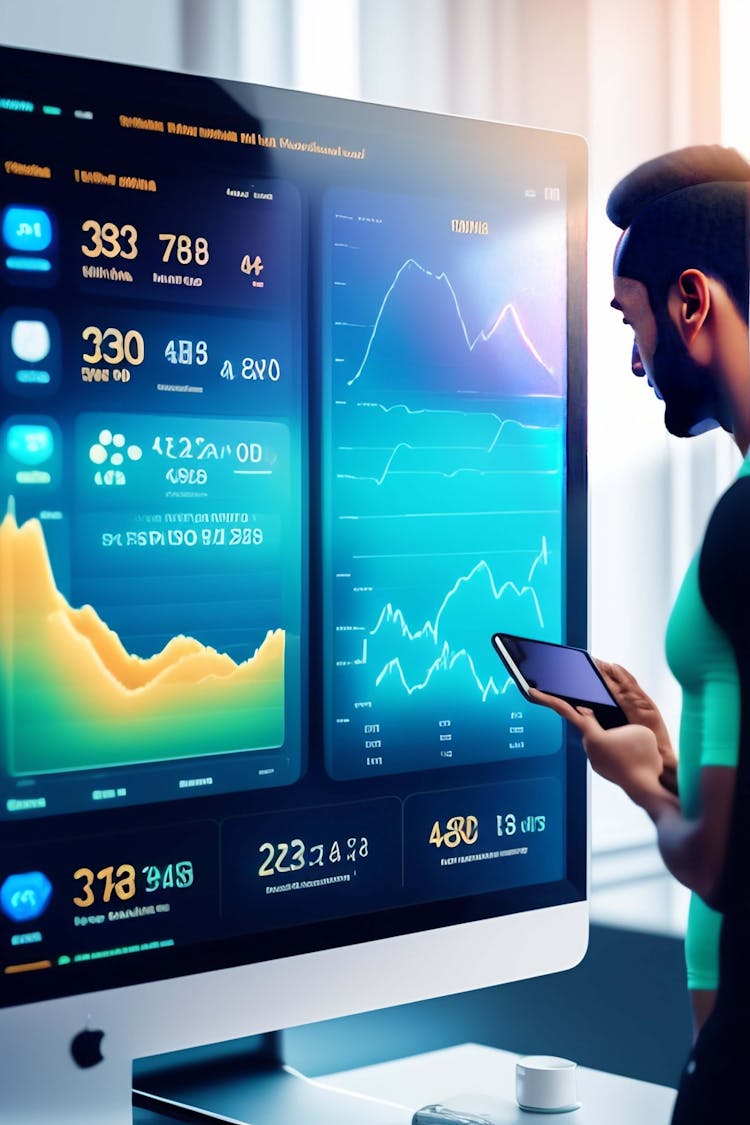
One example of a case study in data science in the e-commerce field is the use of machine learning algorithms to improve product recommendations. In this scenario, an e-commerce company collects data on the browsing and purchasing behavior of its customers.
The company then uses machine learning algorithms to analyze this data and develop a model that can predict which products a customer is likely to be interested in based on their browsing and purchasing history. The model is trained on a large dataset of historical customer data, and is tested and refined to ensure that it is accurate and reliable.
Once the model is finalized, the company can use it to make more personalized product recommendations to its customers. For example, when a customer visits the company's website, the model can recommend products that are likely to be of interest to them based on their previous browsing and purchasing behavior. This can help the company to improve the relevance and quality of its product recommendations, and to provide a better shopping experience for its customers.
In addition, the company can also use the model to identify potential issues or risks, such as customers who are likely to churn or switch to a competitor. By addressing these issues, the company can improve its customer retention and loyalty, and ultimately grow its business.
Overall, this case study shows how data science and machine learning can be used in the e-commerce field to improve the relevance and quality of product recommendations, and to provide a better shopping experience for customers.